AI and Machine Learning in Cybersecurity: How They Enhance Protection
Artificial Intelligence (AI) and Machine Learning (ML) play a significant role in enhancing cybersecurity by
improving threat detection, incident response, and the overall effectiveness of security measures. Here
are some key ways in which AI and ML contribute to cybersecurity:
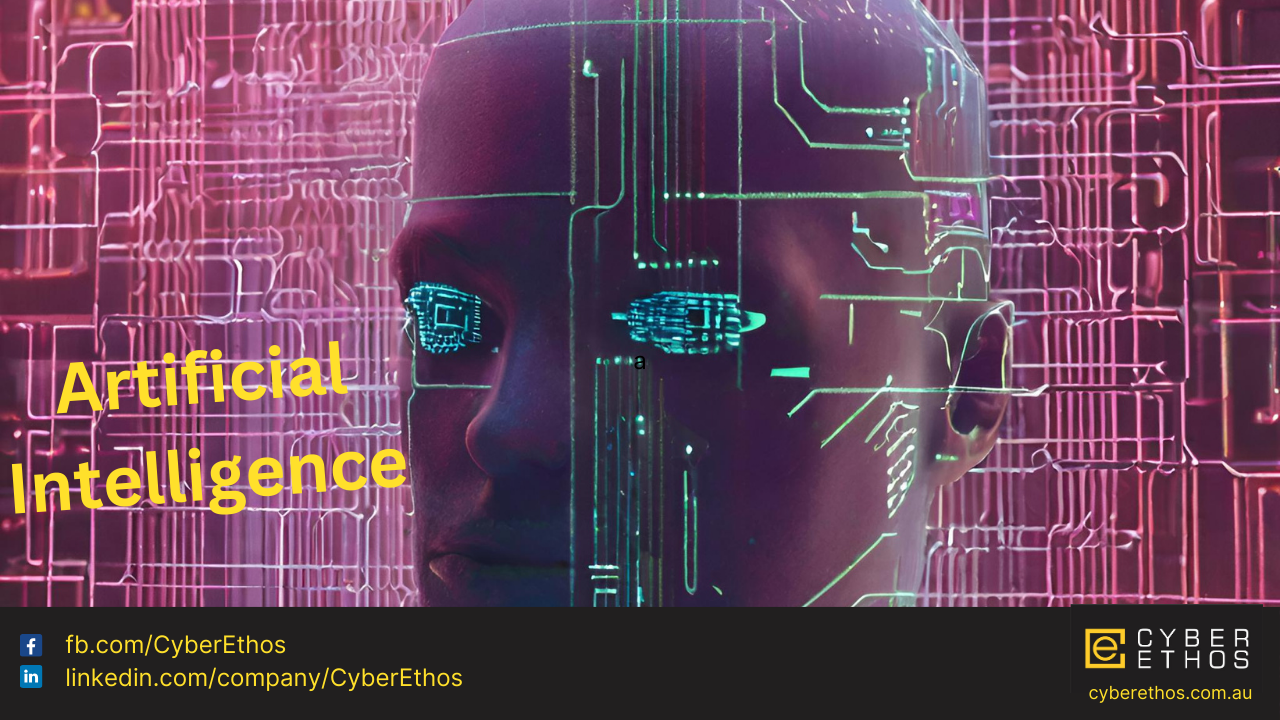
- Threat Detection:
AI and ML systems can analyze massive volumes of data and identify patterns and anomalies that may
indicate cyber threats. They can detect known and unknown threats, such as malware, zero-day exploits,
and insider threats. - Anomaly Detection:
ML algorithms can establish a baseline of normal network or user behavior and raise alerts when
deviations occur. This is particularly valuable for detecting insider threats and zero-day attacks. - Malware and Phishing Detection:
AI-based systems can identify malicious software and phishing attempts by analyzing file attributes,
network traffic, and email content, helping to prevent infections and breaches. - User Behavior Analytics:
ML models can analyze user activities to detect unusual behaviors and potentially compromised
accounts, enhancing the identification of insider threats. - Predictive Analysis:
AI and ML can forecast potential threats and vulnerabilities by examining historical data and threat
intelligence, allowing organizations to proactively strengthen their security posture. - Security Automation:
AI can automate routine security tasks, such as threat triage, to improve response times and free up
human security professionals for more complex tasks. - Adaptive Access Control:
ML algorithms can adapt access controls in real-time based on a user’s behavior and the security
context, reducing the risk of unauthorized access. - Vulnerability Management:
AI-driven systems can prioritize and remediate vulnerabilities by assessing the impact and exploitability
of each security flaw. - Fraud Detection:
In the financial sector, AI and ML are used to detect fraudulent transactions and activities, reducing
financial losses and preventing unauthorized access. - Security Analytics:
AI and ML can sift through vast amounts of data to identify relevant security events, helping security
teams focus on the most critical incidents. - Threat Intelligence:
AI can process and analyze threat intelligence feeds to provide real-time information about emerging
threats, helping organizations take proactive measures. - Cognitive Security:
Cognitive security systems can understand natural language, enabling more effective analysis of security
reports, logs, and threat alerts. - Behavioral Biometrics:
ML can analyze unique patterns in user behavior, such as typing speed, mouse movements, and
touchscreen gestures, to enhance identity verification and detect account compromises. - Chatbots and Virtual Assistants:
AI-powered chatbots and virtual assistants can help users with security-related queries and support,
increasing user awareness and providing assistance in real-time. - Pattern Recognition:
AI can recognize complex patterns in network traffic and system data, aiding in identifying sophisticated
attacks. - Privacy Protection:
ML can help organizations protect user privacy by automatically classifying and securing sensitive data,
such as personally identifiable information (PII). - Zero-Day Vulnerability Identification:
ML models can analyze code and behavior to identify potential zero-day vulnerabilities, enabling early
mitigation. - Network Security:
AI can bolster network security by identifying and mitigating network-based threats, such as Distributed
Denial of Service (DDoS) attacks. - Ransomware Protection:
AI can detect and respond to ransomware attacks more rapidly, potentially mitigating the damage. - Scalability:
AI and ML can scale up to analyze large volumes of data and threats, making them valuable in modern,
data-intensive environments.
While AI and ML can significantly enhance cybersecurity, they are not a silver bullet. They work best
when integrated into a holistic cybersecurity strategy that combines human expertise, well-defined
policies, and robust processes. Additionally, their effectiveness depends on the quality of data, ongoing
tuning, and constant adaptation to emerging threats.